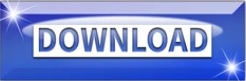
Consequently, there are inaccuracies if microphysics parameterizations are forced with grid box averages of model fields, such as liquid water content. One problem in computing cloud microphysical processes in coarse-resolution numerical models is that many microphysical processes are nonlinear and small in scale. 4, but for the FIRE stratocumulus simulation.
NONUNIFORM LATIN HYPERCUBE SAMPLING PDF
(d) The “true” LES Kessler autoconversion (diamonds) along with analytic approximations based on a binormal PDF (thick solid line), within-cloud liquid water (x marks), and grid box average liquid water (asterisks) the binormal PDF approximation is somewhat in error, and the within-cloud and grid box average liquid water give underestimates, as expected. (c) Time-averaged autoconversion: a Latin hypercube sample ( n = 1, n t = 12) (triangles) is less noisy than traditional Monte Carlo (circles), with no additional calls to the microphysics. (b) The std devs: using two sample points gives lower values, i.e., reduces noise, as desired. (a) An instantaneous snapshot of the estimates: using two sample points per grid box and time step ( n = 2, squares) reduces the noise somewhat. Here n denotes the number of sample points per grid box and time step n t denotes the total number of points in the Latin hypercube sample. (37) and (40) (thick solid line), traditional Monte Carlo ( n = 1, n t = 1) (circles), Latin hypercube sampling with ( n = 1, n t = 12) (triangles), and Latin hypercube sampling with ( n = 2, n t = 12) (squares). Here F Gt( s) is a truncated Gaussian, and C denotes cloud fraction.Įstimates of grid box average Kessler autoconversion for the BOMEX cumulus simulation. Then the cumulative distribution function F G is used to map V into a point X from a single Gaussian PDF.Ī depiction of a single Gaussian PDF, G( s), its corresponding cumulative distribution function, F G( s), and the corresponding cumulative distribution function associated with the cloudy part ( s > 0), F Gt( s). First a point V is chosen randomly from a uniform distribution. All squares are associated with equal probability (figure not drawn to scale).Īn illustration of the inverse distribution function method for a single Gaussian PDF. (c) The third point is selected randomly from within the only square that remains. (b) The second sample point is chosen, and its row and column are excluded (vertical hatching). The square’s column and row (light shading) are excluded when selecting subsequent squares.
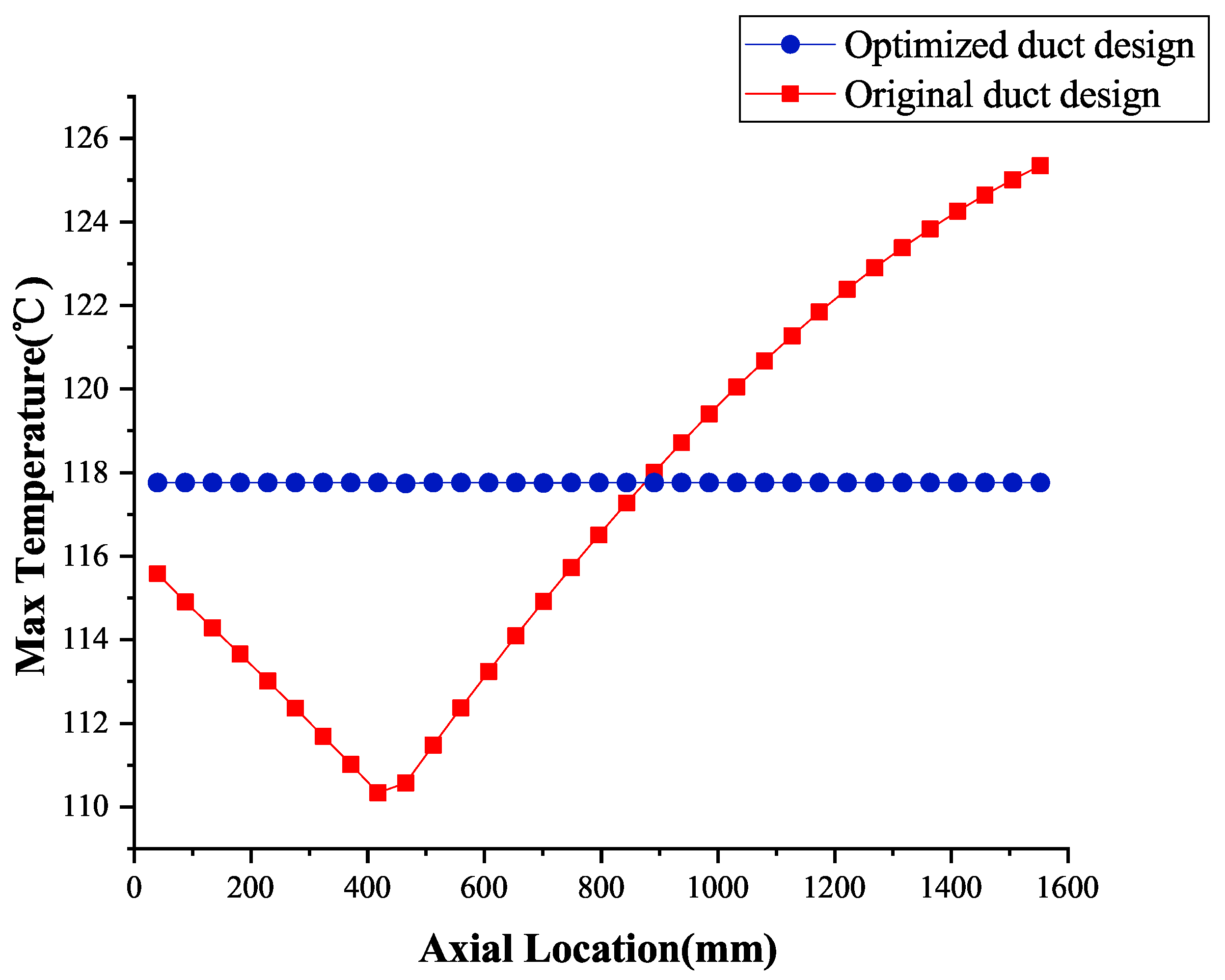

(a) A “square” is chosen at random (dark shading) and within it is randomly chosen the first sample point (dot). The PDF has been divided into three “rows” i.e., strips of nearly constant r t, and three “columns,” i.e., strips of roughly constant w.

A three-point Latin hypercube sample taken from the cloudy portion ( r t > 10) of a bivariate ( w × r t) single Gaussian PDF.
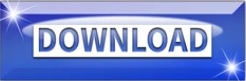